How AI is Revolutionizing Quality Control in Electronics Production
By automating inspections, predicting failures, and optimizing processes, AI is revolutionizing how electronics companies approach quality control.
2/16/20254 min read
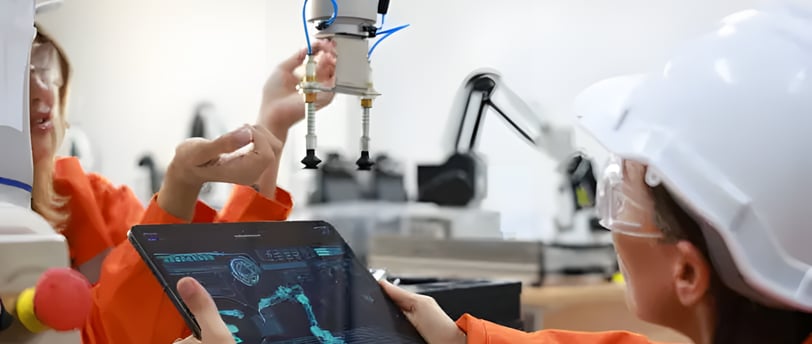
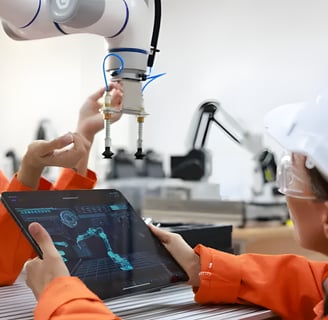
In the fast-paced world of electronics manufacturing, quality control (QC) is a mission-critical process. Whether designing consumer gadgets, industrial electronics, or medical devices, ensuring product reliability and minimizing defects are top priorities. However, traditional QC methods often struggle to keep up with the complexity of modern electronics, leading to inefficiencies, human errors, and high costs.
This is where artificial intelligence (AI) steps in as a game-changer. By automating inspections, predicting failures, and optimizing processes, AI is revolutionizing how electronics companies approach quality control. For designers and decision-makers, understanding how AI-driven QC works—and how it can impact business outcomes—is essential to staying competitive in an industry that demands precision and innovation.
The Role of Quality Control in Electronics Manufacturing
Electronics manufacturing is a high-stakes industry where even the smallest defect can have serious consequences. From faulty microchips causing device failures to poor soldering leading to safety risks, every stage of production needs rigorous quality checks.
Traditionally, QC in electronics relies on visual inspections, functional testing, and statistical process control (SPC). These methods, while effective to a degree, have notable drawbacks:
Human Error: Manual inspections are prone to oversight, especially with intricate circuit designs.
Slow Processes: Conventional testing can become a bottleneck in production lines, slowing time-to-market.
Costly Defect Management: Catching defects late in the process leads to higher scrap rates, rework costs, and warranty claims.
With electronics becoming more compact and complex, traditional QC methods are reaching their limits. This has driven many manufacturers to adopt AI-based solutions that offer more precision, speed, and predictive insights.
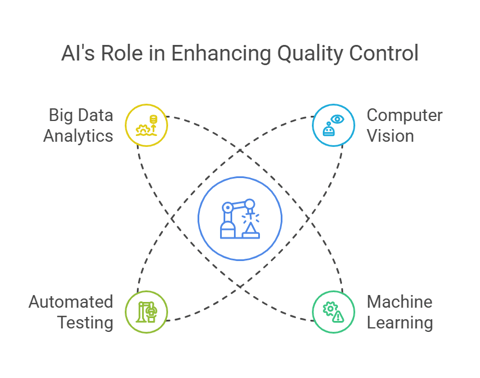
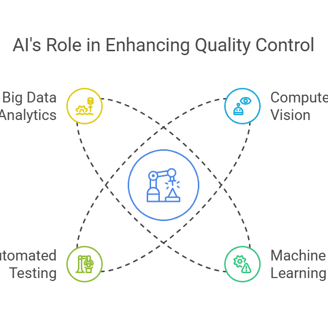
How AI is Transforming Quality Control
AI-driven quality control introduces a new level of intelligence and automation to electronics manufacturing. Here are four key areas where AI is making a significant impact:
1. Computer Vision for Defect Detection
AI-powered image recognition is revolutionizing visual inspections. Using deep learning algorithms, computer vision systems can analyze high-resolution images of electronic components and detect defects that are invisible to the human eye.
For example, AI can inspect solder joints on a PCB (printed circuit board) and identify micro-cracks or misalignments within milliseconds. Traditional optical inspection systems might flag an anomaly, but AI goes a step further by classifying the defect and suggesting corrective actions. This speeds up inspections while reducing false positives and unnecessary rework.
2. Machine Learning for Predictive Maintenance
Equipment failures in electronics manufacturing can cause significant downtime and disrupt production schedules. AI-powered predictive maintenance helps prevent such disruptions by analyzing machine performance data and identifying early warning signs of potential failures.
By continuously monitoring temperature, vibration, and power consumption data, machine learning algorithms can predict when a soldering machine or pick-and-place robot is likely to fail. Instead of relying on fixed maintenance schedules, manufacturers can perform repairs only when necessary, reducing downtime and maintenance costs.
3. Automated Functional Testing & Robotics
Functional testing ensures that each electronic product operates as expected before leaving the factory. AI enhances automated testing by learning from past test results and optimizing testing procedures accordingly.
In high-volume production lines, AI-driven robotic arms can execute precise test sequences on circuit boards, detecting inconsistencies in electrical signals or component behavior. Over time, AI systems refine their analysis, improving test accuracy and efficiency.
4. Big Data & Process Optimization
Manufacturing generates vast amounts of data, from sensor readings to inspection reports. AI-driven analytics turn this data into actionable insights, helping manufacturers optimize QC processes.
For instance, AI can analyze production data across multiple assembly lines to identify patterns that lead to defects. If a certain batch of components consistently fails in a specific condition, AI can pinpoint the root cause—whether it’s a supplier issue, a temperature fluctuation, or a misconfigured machine setting. This empowers manufacturers to make data-driven decisions to improve yield and minimize defects.
Key Benefits of AI in Quality Control
The adoption of AI in QC brings tangible benefits to electronics manufacturers, including:
Higher Accuracy: AI eliminates human errors in defect detection, ensuring more consistent quality.
Faster Inspections: AI-driven vision systems analyze thousands of components per second, accelerating production speed.
Cost Reduction: AI minimizes scrap, rework, and warranty claims by catching defects early.
Regulatory Compliance: AI-driven traceability ensures adherence to industry standards such as IPC, ISO, and UL certifications.
Enhanced Process Optimization: AI continuously improves QC procedures, reducing long-term operational costs.
Real-World Applications & Case Studies
Many electronics manufacturers are already seeing significant gains from AI-driven quality control. For example, a global semiconductor company implemented AI-based defect detection in its wafer fabrication process. By analyzing thousands of wafer images in real-time, AI reduced defect rates by 30% and saved millions in scrap costs.
Another case involves a leading smartphone manufacturer using AI-powered predictive maintenance for its SMT (surface-mount technology) machines. By preventing unplanned downtime, the company improved production efficiency by 25% while extending the lifespan of critical equipment.
Challenges & Considerations in Implementing AI for QC
Despite its advantages, adopting AI for quality control comes with challenges:
High Initial Investment: AI-driven QC systems require upfront investment in hardware, software, and training.
Integration Complexity: AI solutions must be seamlessly integrated into existing manufacturing processes without causing disruptions.
Need for Skilled Personnel: AI-driven QC requires expertise in data science, machine learning, and manufacturing operations.
Data Security Concerns: AI systems rely on vast amounts of sensitive production data, requiring robust cybersecurity measures.
For companies considering AI-driven QC, a phased approach—starting with pilot projects before scaling up—can help mitigate these challenges and ensure a smoother transition.
Future Trends in AI-Powered Quality Control
As AI technology continues to evolve, several exciting trends are shaping the future of quality control in electronics production:
Deep Learning for Ultra-Precision Inspections: AI models are becoming more sophisticated, capable of detecting even the slightest imperfections.
AI-Powered Self-Healing Systems: Future production lines may feature AI that not only detects defects but also autonomously adjusts processes to correct them in real time.
Integration with IoT & Industry 4.0: AI-driven QC will increasingly work alongside connected devices, enabling fully automated and intelligent manufacturing environments.
Conclusion
AI is transforming quality control in electronics manufacturing from a reactive process to a proactive, intelligent system that prevents defects before they occur. For designers and decision-makers, embracing AI-driven QC is no longer a luxury but a necessity to remain competitive in an industry where precision and efficiency define success.
By leveraging AI for defect detection, predictive maintenance, automated testing, and process optimization, electronics companies can achieve higher product reliability, faster time-to-market, and substantial cost savings. While the road to AI adoption comes with challenges, the long-term benefits far outweigh the risks.
As AI technology advances, those who integrate it into their quality control processes today will be best positioned to lead the electronics industry of tomorrow.